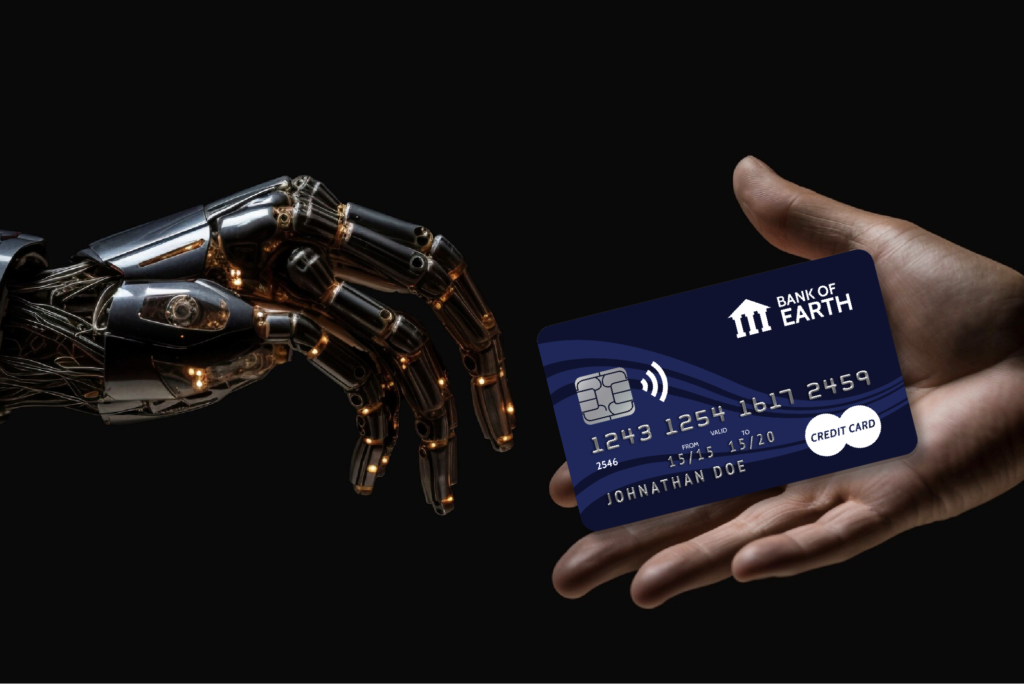
What is Generative AI?
The evolution of machine learning models and the introduction of Generative AI (”GenAI”) will present the largest impact on mitigating the impact of fraud in payments in recent history.
Machine learning models in its simplest forms have been employed in the banking industry, technology, and other sectors since the 1950s. However modern ML architectures allow for an ever increasing number of features to be utilized, in combination with neural networks and external data points, to provide better predictions and increase detection capabilities.
Unlike legacy models, GenAI is more robust and has better continuous learning capabilities, is able to adapt to new fraud trends faster, and has already demonstrated an increased ability to identify false positives. GenAI models can be deployed more quickly within fraud risk engines, while learning from observed patterns to better distinguish legitimate activity. GenAI can also provide operational support by providing predictive recommendations and insights to help human agents make better decisions in their fraud reviews.
How does Generative AI impact fraud?
GenAI is Increasing the Sophistication of Attacks:
How AI Is Enhancing Enumeration Attacks
Fraud actors are leveraging GenAI in order to create synthetic identities that closely mimic legitimate consumer behavior and demographics. We have observed this in enumeration attacks: traditionally such attacks were conducted using simple scripts, with the same BIN/card number utilized, in low dollar amounts, and with easy to identity fake cardholder data. Modern enumeration attacks are characterized by a high degree of sophistication: each authorization attempt presents different cardholder details, amounts attempted vary with each transaction, and timing of attacks is spaced across days and weeks in order to reduce the likelihood of detection.
The Rise of Forged Documents in KYC/KYB
AI has allowed fraud actors to create forged documentation and other visual references, which increase the complexity of KYC/KYB validations, and often times pass simple verifications performed my many of the vendors active in the industry. By training on thousands of available records of drivers licenses, business licenses, and other financial documents, GenAI has been able to mimic such documents to a high degree, often times resulting in human review and analysis. Such assessments are not feasible for marketplaces, aggregators, and other industries that conduct KYC at scale, and significant investment is already being invested in developing technologies (many AI driven) in order to help improve automated detection techniques.
AI Voice Cloning and Social Engineering Scams
Fraud rings have also been able to utilize easily available AI tools in order to increase the sophistication of social engineering scams. One of the most prevalent type of scams is the “caller in distress” scenario – fraud actors use AI in order to capture voice bytes from their victims, and create fake phone calls to their relatives, defrauding victims of significant amounts of money in a short amount of time. Recent research suggests that even 3 seconds of audio data capture may allow fraud actors to replicate a victim’s voice, an unparalleled advancement in fraud technology. The AI voice cloning technology is also being utilized across payments – to target banks, customer support centers of merchants, and even fintechs for a variety of purposes: gaining access to financial records, funding, PII data, and also in order to conduct account take overs (”ATO”).
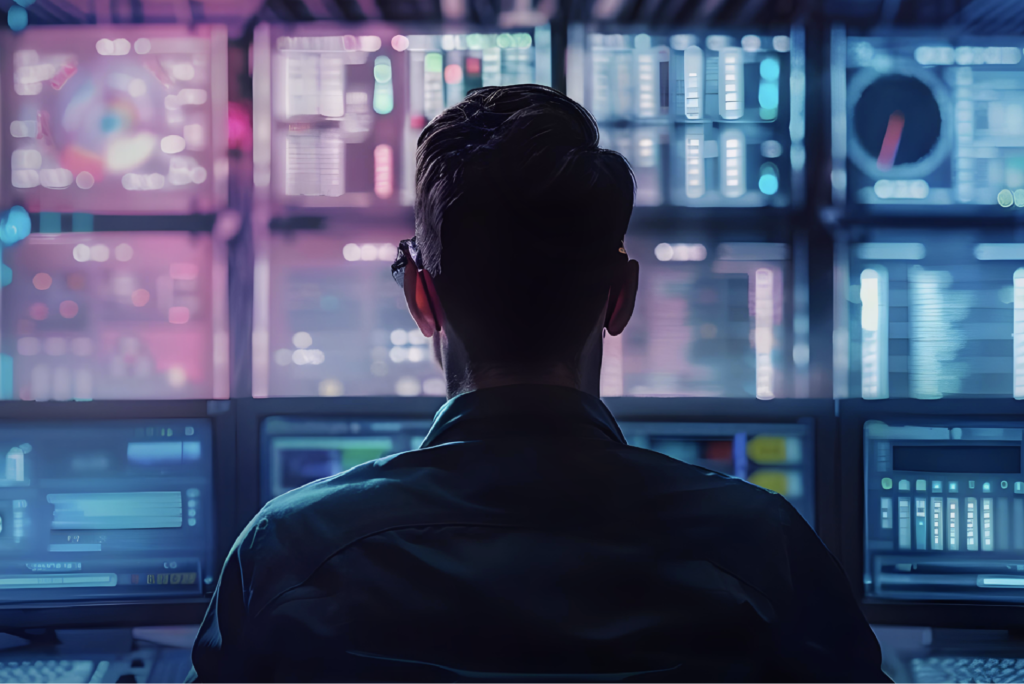
GenAI is Increasing the Speed and Scale of Fraud Events
The availability of GenAI solutions to fraud actors has allowed attacks to be deployed quickly, and extensively across payment networks, financial institutions, and merchant accounts. Unlike traditional scripts, or manual fraud attack methodologies (typing an order information in a website’s checkout page, etc.), AI has allowed fraud actors to create simple prompts that create entire lists of cardholder data points, scripts that allow mass data to be submitted in checkout flows in one click, and has in general allowed the creation of sophisticated “BOTs” that have increased the operational efficiency of fraud rings to a magnitude unseen in the past. An example of the impact of such increases in scale is in enumeration – card networks in the US predict that over $1 Billion was lost by merchants in 2024 due to card testing attacks.
How Can FinTechs and Merchants Combat AI Fraud?
The good news for payment companies and merchants is that GenAI (and AI technology in general) is also available to them to fight Fraud. Open source AI models (such as the ones OpenAI offers) can allow businesses to leverage existing model capabilities in order to build a custom AI applications.
AI applications can offer significant improvements over traditional models:
- GenAI models can analyze vast amount of data in order to identify patterns and anomalies that human analysts might miss. Traditionally, fintechs and merchants have employed rule based solutions, and leveraged case management for payments monitoring. Such solutions offer operational inefficiencies, result in high operating costs, and often can result in a high amount of false positives being assessed. Simple GenAI applications can serve as primary point of assessment, and only trigger a step up escalation, when the models detect an anomaly based on historical patterns.
- GenAI can offer improved predictive recommendation sand insights, helping human agents make better decisions in real-time. AI applications can be trained on historical human review data, leveraging approve-decline decisions in combination of historical order and payments data, in order to provide, not just, initial screening, but also predictive recommendations for action.
- Open source technologies also allow small companies to minimize the amount of investment required in order to build AI applications. Traditional machine learning models required significant investment in engineering, data science, and other areas, as there has always been a taxing requirement on support infrastructure to support model development. AI allows small teams (often times led by one data scientist) to leverage AI frameworks trained on proprietary data, in order to build analytic and decisioning solutions in a time frame that is 10x lower than that of traditional models, and for a fraction of the cost.
The Importance of Governance
As companies scope the utilization of GenAI in application building, it’s extremely important to consider data protection and governance. Some open source AI models require data sharing, resulting in potential privacy clashes with existing regulation and internal policies. It’s essential for risk and fraud teams to partner with their information security counterparts in order to assess data sharing and retention of potential AI partners. Organizations should ensure that they create and enforce data usage, storage, and retention policies, and that all AI applications would fall within accepted use cases.
Share this Article